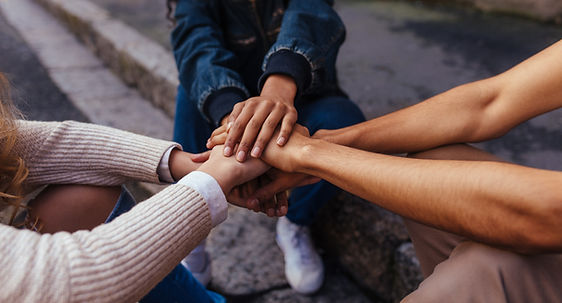
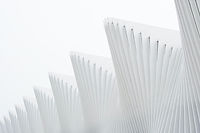
Introduction to university rankings
A variety of associations and organizations have developed formulas and algorithms to rank the performance, status, or capabilities of universities across the globe. For example, one of the pioneers is the Academic Ranking of World Universities or ARWU, first developed by by Shanghai Jiao Tong University in 2003. To rank universities, the Academic Ranking of World Universities considers four key features
-
quality of education, as measured, perhaps controversially, by the number Nobel laureates & Fields Medalists
-
quality of academics, as measured by the number of researchers that are highly cited, according to the Thomson Reuters survey, and the number of staff with Nobel laureates & Fields Medalists
-
research output, as measured by the number of publications in Nature and Science and number of publications, according to the Science Citation Index-expanded and Social Science Citation Index
-
a weighted average of the previous indicators divided by the number of academics
Some ranking schemes integrate more information, including subjective perceptions. To illustrate, the QS World University Rankings evaluates
-
reputation from academics around the world in each discipline, called academic peer review, in which scholars complete surveys about their perceptions of an institution—weighted 40% of the overall rank
-
ratio of staff to students
-
number of times university publications are cited—divided by the number of research-active staff
-
attitudes of employees towards the graduates of this institution
-
the percentage of students and the percentage of staff who are international
The Times Higher Education World University Rankings, produced by the Times Higher Education magazine, is similar to the QS World University Rankings but is more dependent on research and teaching income. Specifically, this ranking integrates measures such as
-
research income from industry and teaching income from students
-
the percentage of students and the percentage of staff who are international
-
number of PhD completions and undergraduate students per academic
-
reputation of teaching and research, as derived from a survey of peers
-
number of publications per academic or researcher and number of citations per publication, weighted to control academic disciplines
This ranking assigns the most weight to the surveys to gauge reputation of teaching and research and the number of citations per publication. Appendices 1, 2, and 3 display more information, adapted from Vernon et al. (2018), on the research metrics, IP metrics, and teaching measures that correspond to the major ranking schemes. Institutions can utilize this information to decide which metrics to prioritize, depending on the ranking schemes they value.
Of course, all the ranking algorithms have attracted some criticism. As Rhein and Nanni (2021), scholars tend to criticize the competitive ideology these rankings presuppose, the inadvertent effects of these rankings on the decisions of university leaders and other stakeholders, as well as the methods these schemes utilize. To illustrate a criticism of the methods, according to Docampo and Cram (2015), about 30% of the variance in the ARWU ranking can be attributed to the size of institutions instead of the quality (see also Cano et al., 2018)
Applications of university rankings: Overview
Although university rankings have been criticized frequently and forcefully, many stakeholders depend on these rankings to reach a variety of decisions (Johnes, 2018; Vernon et al., 2018). For example
-
students frequently apply to universities that are ranked higher over a university that is ranked lower
-
academics in particular, and staff in general, often choose to work at universities that are ranked highly
-
marketing campaigns often refer to their ranking, if sufficiently high, to attract students, staff, industry partners, and other stakeholders
-
governments often assess the rankings of universities in their state or nation to evaluate past initiatives
-
university leaders often consider their rankings to adapt or to transform their strategies and practices
-
industries sometimes consider the research performance and quality of universities to identify a research collaborator.
The degree to which stakeholders depend on rankings to reach decisions varies across countries, communities, and circumstances. To illustrate, at least historically, rankings were perceived as more legitimate in some nations, such as the US and UK, than other nations, such as many European countries. In particular, rankings are perceived as more valuable in nations that value competition between institutions compared to nations that prefer more uniformity across institutions (Horstschräer, 2012; Koenings et al., 2020).
Applications of university rankings: Choice of universities in which to study
Research does indeed confirm that university rankings do shape decisions of domestic students and international students on where to study (see Griffith & Rask, 2007). To illustrate, Koenings et al. (2020) administered a survey to over 500 Masters students, enrolled at a German university. The survey included a series of questions about the qualities that students considered when choosing a university as well as the academic background and discipline of the students. The data were subjected to a factor analysis. The factor analysis uncovered four key factors in domestic students and four other factors in international students that determine the university in which these individuals will enroll. Specifically, domestic students often considered
-
living conditions, such as availability of affordable accommodation, quality of life, as well as costs of living and tuition
-
reputation and ranking of the university
-
personal motives, such as distance from the family home
-
suitability of the course and teaching
In contrast, international students often considered
-
ranking, reputation, and suitability of the course and teaching
-
living conditions and reasonable admission requirements
-
strength of the international student community
-
personal motives, such as distance from the family home
Accordingly, in both domestic students and international students, ranking is considered but only a facet of one or four key determinants of these choices. Yet, a variety of characteristics affect the degree to which students consider the ranking of universities during their choice of where to study. Specifically, as an additional multiple regression analysis revealed, domestic students are more likely to consider university rankings if their friends had also imparted relevant insights about the various universities. International students are more likely to consider rankings if their teachers had not imparted relevant insights about the various universities.
Conceivably, the association between university rank and attraction of students might not be linear. To illustrate, if the rank of a university is especially high, the institution might appear on prestigious lists (Bowman & Bastedo, 2009), such as list of the top 50 universities. If the rank of a university is not especially high and the institution does not appear on these prestigious lists, further declines may not decrease student attraction to the same extent.
Other studies, however, indicate that university rankings may not affect which university applicants will choose, at least in some circumstances. To illustrate, Soo (2013) explored whether league tables, published in the Sunday Times University Guide, designed to rank UK universities, affects the decisions of students. They revealed that league tables did not significantly influence the university to which potential students apply. Instead, recommendations from friends and family—and insights from alumni of a university—were more likely to shape their decisions.
'
Applications of university rankings: Google searches
Rather than explore the association between university rankings and student attraction, some universities have examined whether these rankings influence popularity, as measured by the number of Google searches or similar metrics (e.g., Taylor & Bicak, 2020). Rybiński and Wodecki (2022), for example, showed that QS ranking scores are positively associated with the Google Search Volume Index, a measure of how often users search this institution. This pattern was observed in a range of countries—except perhaps China, where Google searches are restricted. Nevertheless, small improvements in the QS ranking did not increase Google searches in the next year or two.
Applications of university rankings: World Universities Ranking Simulator or WURS
Siniksaran and Satman (2019) developed software, called WURS, that enables individuals, such as researchers or university leaders to explore and to utilize university rankings more efficiently. Typically, stakeholders can access only the overall ranking of a university in each scheme. For example, they might discover that a particular university is ranked 100 by THE, 150 by QS, and 200 by ARWU. They are not usually informed of how the university performed on each metric, such as number of patents, relative to competitors.
WURS was designed to address this limitation. That is, this software enables the user to estimate the performance of an institution and thus ascertain strategies that could improve the THE, QS, and ARWU rankings in particular. In particular, users merely need to enter the raw scores on various metrics, such as the number of publications. WURS can then estimate the performance of this institution on the metric, relative to other universities, from a series of nonlinear regression equations.
Limitations of rankings: Information that diverges from priorities of students
When choosing between universities, students do consider rankings (Koenings et al., 2020). Yet, the ranking of universities primarily depends on parameters that students do not value. To illustrate, in many circumstances, students tend to prefer universities in which
-
significant resources are dedicated to teaching (Drewes & Michael, 2006)
-
student services are excellent, especially services that are not related to study (Drewes & Michael, 2006)
-
the number of students in each class is small (Drewes & Michael, 2006)
-
research activity is limited (Drewes & Michael, 2006)
-
tuition is not too expensive
Yet, universities that are ranked highly are not as likely to provide these qualities. Universities that are ranked highly tend to invest significantly into research, for example. Likewise, universities that are highly ranked often charge steep tuition fees.
Limitations of rankings: Biased measures
To rank universities, many of the schemes administer surveys to academics, inviting these individuals to evaluate the research, teaching, and commercial reputation of a university. Indeed, most ranking schemes, including the THE and QS, depend on these subjective measures.
Indeed, as Safón and Docampo (2020), even metrics that might seem objective may be biased by the reputation of institutions. To illustrate, as these researchers demonstrated, the prestige of an institution affects the likelihood that manuscripts are published in Nature or Science. Consequently, a vicious cycle may unfold: If the quality of an institution is overrated, more of the manuscripts this institution produces will be accepted in Nature or Science rather than rejected. Consequently, the ARWU—a scheme that is heavily dependent on the number of publications in Nature or Science—may inflate the ranking of this institution. Because of this inflated ranking, even more of the manuscripts this institution generates will be accepted in Nature or Science, and so forth.
Limitations of rankings: Inconsistency across rankings
Unsurprisingly, the various ranking schemes can generate conflicting information. Vernon et al. (2018) presented a case study to illustrate these conflicts. In this case, 10 rankings schemes had evaluated the research performance of one university. The ranks varied considerably. For example, Thomson Reuters Innovative University Ranking indicated the research performance of this university was in the top 25. In contrast, University Rankings by Academic Performance indicated the research performance of this university was outside the top 120. This variability indicates that
-
in marketing campaigns, universities can often identify one ranking scheme to defend their reputation even if most ranking schemes generate conflicting results
-
the reliability and validity of these schemes is thus questionable.
Some researchers, however, have suggested these concerns may be overstated. Shehatt and Mahmood (2016), for example, revealed moderate to high correlations across six of the most common university ranking schemes. These correlations are especially high when the analysis examines 200 or more universities.
Limitations of rankings: Other concerns
Scholars have raised many other concerns about these university rankings schemes. Specifically
-
some of the metrics that influence the rankings do not represent the quality of ongoing education or research, such as number of Nobel-prizewinning alumni
-
most of the rankings do not assess the degree to which universities fulfill their missions and improve society. The rankings seem to prioritize research and commercialization over teaching and societal benefits.
Adverse effects of rankings: Competition
Many scholars express the concern that university rankings elicit a competitive mindset that may ultimately stifle collaboration, diminishing the likelihood innovative ventures that resolve enduring problems in society (see Brankovic et al., 2018). To illustrate, as Brankovic et al. (2018) argues that, because university rankings have become so entrenched and significant, university leaders have developed a mindset in which they prioritize quantitative comparisons and perceive the success of a rival as a failure in their own institution: a zero-sum game. Even reputation is regarded as scarce. So, institutions become disinclined to enhance the reputation of one another.
Similarly, according to Brankovic et al. (2018), university rankings inculcate a notion of global excellence. Specifically, implicit in the pursuits of university leaders is the assumption that institutions should strive to achieve some universal state of excellence. However, many counterarguments challenge the legitimacy of this assumption. To illustrate
-
this belief in global excellence deviates from the recognition that all institutions develop their own mission—a mission that is germane to their distinct circumstances—and should strive to accomplish this mission
-
this belief in global excellence also implies the motivations and qualities of dominant universities now, such as the pursuit of research income, are inherently commendable.
-
this belief in global excellence implies that quantitative metrics, such as research output, are equally valid measures of beneficial qualities in all nations and communities
-
this belief in global excellence assumes all audience, such as students or staff in all nations, benefit from the same attributes
Furthermore, as Brankovic et al. (2018) reveal, university rankings tend to demonstrate, and may even inflate, the level of flux in the sector. And this flux can instill a sense of instability that amplifies competition. To illustrate
-
even minute or random changes in organizations can significantly shift the rank of a university from the top of a table, as displayed on a webpage, to the bottom.
-
some changes in ranking actually emanate from updates to the formulas the various schemes utilize—common in QS World University Rankings and Times Higher Education World University Rankings
-
many of the ranking schemes are media companies and thus like to inflate change to attract global attention.
Adverse effects of rankings: Inequality
Rankings may actually entrench and indeed exacerbate the inequalities of resources across institutions (Baltaru et al., 2022). In essence, if the resources of a university are limited—perhaps because of hardships or challenges the institution cannot prevent or control—research, commercial, and teaching performance might subside. The ranking of this institution may thus subside. Because of this decrease in the ranking, the university, especially if not prestigious, may attract fewer students. Resources will thus decline further, potentially igniting an inexorable cycle of problems.
Baltaru et al. (2022) indeed substantiated this possibility, analyzing data collected about 102 UK universities over 10 years. The authors conducted linear regression, exploring the association between ranking position and financial sustainability—the ratio of surplus to deficits—after controlling institutional size and previous financial sustainability. The key finding was that decreases in university ranking diminished financial sustainability, but only in universities that were not ranked in the top echelon.
Other effects of rankings: Strategic change
Unsurprisingly, as university rankings became increasingly prominent, institutions began to shift their strategies to prioritize activities that optimize these rankings, often successfully. Dowsett (2020) presents a detailed analysis of how four Australian universities—Curtin University, Deakin University, QUT, and Wollongong University—adapted their strategies in response to dissatisfaction with their rankings, from 2003 to 2020. For example
-
some of the universities explicitly encouraged staff to publish in Nature and Science: a key metric of the Academic Ranking of World Universities or ARWU
-
strategic plans and complementary papers displayed rankings and showed how specific initiatives did indeed shape some of these rankings.
-
the universities became more inclined to celebrated academics who were highly cited
-
the universities often introduced schemes to attract professors who were highly cited, sometimes called iconic scholars.
-
the universities pledged to align research initiatives to ranking metrics
-
one university introduced an accelerated PhD program
Consequently, or perhaps coincidentally, all four universities achieved improvement on the ARWU.
Evaluations of rankings
To reiterate, many commentators have discussed the limitations of university rankings as well as the adverse effects of these schemes. In response, some researchers have designed more systematic methods to evaluate these rankings.
Gadd (2020) outlined an intriguing example. A team of researchers distilled a range of criteria from the literature but then sought feedback from academics, research professionals, and other stakeholders about these criteria. This feedback was reduced to 20 principles that ranking schemes should follow. To illustrate, according to these principles, university ranking schemes should
-
declare financial conflicts of interest
-
transparently communicate the aims of their schemes as well as the methods and data they utilize to rank universities
-
measure qualities that are compatible with the mission of each university
-
generate rankings that match the qualities they purport to measure
-
assess the degree to which the universities conduct activities that fulfill societal needs, such as equality, diversity, open access, and sustainability
The team then designed a tool that assesses the degree to which the various ranking schemes conform to these principles (for more information, see https://inorms.net/research-evaluation-group/). Six international specialists applied this tool to evaluate six ranking schemes. The CWTS Leiden Ranking scheme also agreed to evaluate themselves on this tool.
In general, these ranking schemes—such as Academic Ranking of World Universities, QS World University Rankings, Times Higher Education World University Rankings, and US News & World Report global ranking—did not conform to some of the key principles. For example
-
the qualities did not greatly match the qualities they purport to measure
-
they did not tend to measure qualities that are compatible with the mission of each university
-
they did not measure the degree to which universities conduct activities that fulfill societal needs, such as equality, diversity, open access, and sustainability
Similarly, Vernon et al. (2018) also evaluated a set of 13 ranking schemes on a set of criteria. These researchers, however, were more interested in the degree to which the rankings schemes may enable universities to identify and to address shortfalls in their research. Therefore, the criteria were more relevant to research and included
-
the degree to which the ranking integrated a comprehensive range of characteristics, such as intellectual property
-
the extent to which the ranking depends on objective, empirical data rather than self-reported data or surveys
-
the degree to which the calculation methods and the data that were used are transparent
This analysis revealed a range of shortcomings. To illustrate
-
to evaluate the quality of research, many rankings depend heavily on research expenditure; yet, studies indicate a low, and sometimes negative, correlation between research expenditure and research quality (e.g., Bowen & Casadevall, 2015; Mongeon et al., 2016)
-
some rankings, according to Vernon et al. (2018), were too reliant on peer reputation. Peer reputation is biased towards larger universities.
-
almost half the rankings depended on some level of self-report
References
-
Baltaru, R.-D., Manac, R.-D., & Ivan, M.-D. (2022). Do rankings affect universities’ financial sustainability? Financial vulnerability to rankings and elite status as a positional good. Studies in Higher Education
-
Bowen, A., & Casadevall, A. (2015). Increasing disparities between resource inputs and outcomes, as measured by certain health deliverables, in biomedical research. Proceedings of the National Academy of Sciences, 112(36), 11335-11340.
-
Bowman, N. A., & Bastedo, M. N. (2009). Getting on the front page: Organizational reputation, status signals, and the impact of US News and World Report on student decisions. Research in Higher Education, 50(5), 415-436.
-
Brankovic, J., Ringel, L., & Werron, T. (2018). How rankings produce competition: The case of global university rankings. Zeitschrift für Soziologie, 47(4), 270-288.
-
Cano, A. F., Marin, E. C., Rodrigues, M. T., & Ruiz, M. V. (2018). Questioning the Shanghai Ranking methodology as a tool for the evaluation of universities: An integrative review. Scientometrics,116(3), 2069–2083.
-
Clarke, M. (2007). The impact of higher education rankings on student access, choice, and opportunity. Higher Education in Europe, 32(1), 59-70.
-
Docampo, D., & Cram, L. (2015). On the effects of institutional size in university classifications: The case of the Shanghai ranking.
-
Dowsett, L. (2020). Global university rankings and strategic planning: a case study of Australian institutional performance. Journal of Higher Education Policy and Management, 42(4), 478–494
-
Drewes, T., & Michael, C. (2006). How do students choose a university? An analysis of applications to universities in Ontario, Canada. Research in Higher Education, 47(7), 781-800.
-
Fauzi, M. A., Tan, C. N. L., Daud, M., & Awalludin, M. M. N. (2020). University rankings: A review of methodological flaws. Issues in Educational Research, 30(1), 79-96.
-
Gadd, E. (2020). University rankings need a rethink. Nature, 587(7835), 523-524.
-
Griffith, A., & Rask, K. (2007). The influence of the US News and World Report collegiate rankings on the matriculation decision of high-ability students: 1995–2004. Economics of Education Review, 26(2), 244-255.
-
Horstschräer, J. (2012). University rankings in action? The importance of rankings and an excellence competition for university choice of high-ability students. Economics of Education Review, 31(6), 1162-1176.
-
Johnes, J. (2018). University rankings: What do they really show? Scientometrics, 115(1), 585-606.
-
Koenings, F., Di Meo, G., & Uebelmesser, S. (2020). University rankings as information source: Do they play a different role for domestic and international students? Applied Economics, 52(59), 6432-6447.
-
Mongeon, P., Brodeur, C., Beaudry, C., & Larivière, V. (2016). Concentration of research funding leads to decreasing marginal returns. Research Evaluation, 25(4), 396-404.
-
Rhein, D., & Nanni, A. (2021). The impact of global university rankings on universities in Thailand: don’t hate the player, hate the game. Globalisation, Societies and Education.
-
Rybiński, K., & Wodecki, A. (2022). Are university ranking and popularity related? An analysis of 500 universities in Google Trends and the QS ranking in 2012-2020. Journal of Marketing for Higher Education.
-
Safón, V., & Docampo, D. (2020). Analyzing the impact of reputational bias on global university rankings based on objective research performance data: the case of the Shanghai Ranking (ARWU). Scientometrics, 125(3), 2199–2227.
-
Saisana, M., D’Hombres, B., & Saltelli, A. (2011). Rickety numbers: Volatility of university rankings and policy implications. Research Policy, 40(1), 165–177.
-
Shehatta, I., & Mahmood, K. (2016). Correlation among top 100 universities in the major six global rankings: Policy implications. Scientometrics,109(2), 1231–1254.
-
Siniksaran, E., & Satman, M. H. (2019). WURS: A simulation software for university rankings—software review. Scientometrics, 122(1), 701–717.
-
Soo, K. T. (2013). Does anyone use information from university rankings? Education Economics, 21(2), 176-190.
-
Vernon, M. M., Balas, E. A., & Momani, S. (2018). Are university rankings useful to improve research? A systematic review. PloS one, 13(3).
.Appendix 1: Degree to which common ranking schemes depend on various research metrics. Note, numbers represent relative weights.
Measures | ARWU | CA | CWUR | Lieden | QS | SCIMago | THE | UMR | URAP | USN-WN |
---|---|---|---|---|---|---|---|---|---|---|
Number of scientific articles | Yes | 10 | 5 | |||||||
Number of publications | Yes | Yes | Yes | Yes | 8 | 6 | Yes | 21 | 10 | |
Number of citations, normalized | Yes | Yes | Yes | Yes | Yes | 13 | 30 | Yes | 21 | 7.5 |
Number of articles as corresponding author | 5 | |||||||||
Number of publications in top journals | Yes | Yes | 2 | |||||||
Number of publications with external collabators | Yes | |||||||||
Number of publications with international collaborators | Yes | 2 | 2.5 | Yes | 15 | 10 | ||||
Number of publications with industry collaborators | Yes | Yes | ||||||||
Percentage of articles that were the top most cited | Yes | 2 | Yes | 15 | 32.5 | |||||
Percentage of articles that were the top most cited as main contributor | 13 | |||||||||
Number of different authors from an institution | 5 | |||||||||
Ratio of citations per publication | 18 | 10 | ||||||||
Interdisclinarity of publications | Yes | |||||||||
Industry article citation | Yes |
.Appendix 2: Degree to which common ranking schemes depend on various intellectual property metrics. Note: numbers represent relative weights.
Measures | ARWU | CA | CWUR | Lieden | QS | SCIMago | THE | UMR | URAP | USN-WN |
---|---|---|---|---|---|---|---|---|---|---|
Patents filed | 11 | 5 | Yes | |||||||
Patents awarded | 11 | |||||||||
Patents filed globally | 11 | Yes | ||||||||
Number of publications cited in patent applications | 11 | Yes | ||||||||
Joint patents with industry | Yes | |||||||||
Start-ups initiated | Yes | |||||||||
R&D expenditure | Yes | |||||||||
R&D from industry | Yes | |||||||||
Publications per research income | 2 | |||||||||
Research income per staff and students | 6 | |||||||||
Science and engineering staff | ||||||||||
Ratio of R&D to institutional income | 2 | |||||||||
Non Science and engineering as well as R&D staff | ||||||||||
Reputation survey about IP | 8 | 25 | ||||||||
Total number of academics | ||||||||||
Nobel prize and field awards in academics or alumni | Yes | 11 | 50 |
.Appendix 3: Degree to which common ranking schemes depend on various teaching measures. Note: numbers represent relative weights.
Measures | ARWU | CA | CWUR | Lieden | QS | SCIMago | THE | UMR | URAP | USN-WN |
---|---|---|---|---|---|---|---|---|---|---|
Reputational survey of academic quality | 40 | 15 | Yes | Yes | ||||||
Income to the institution | 2.5 | |||||||||
Ratio of staff to academics | 20 | 4.5 | Yes | |||||||
Reputation survey from employers | 10 | |||||||||
Number of doctoral degrees | 2.25 | Yes | ||||||||
Number of doctoral degrees per admitted candidate | ||||||||||
Number of doctoral degrees per staff | ||||||||||
Proportion of academics with doctorates | 5 | 6 | Yes | |||||||
Proportion of international students | 5 | 2.5 | Yes | |||||||
Proportion of international staff | 2.5 | Yes | ||||||||
Number of international students in Bachelor programs | ||||||||||
Number of alumni who are CEOs in Forbes 100 | 25 | |||||||||
Web presence | 5 | |||||||||
Inbound links to web | 15 | |||||||||
Staff per number of Bachelor students | ||||||||||
Number of doctoral students divided by number of Bachelor students |
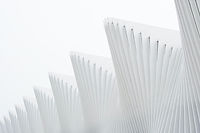
Random grant allocation
Introduction: Peer review
In recent years, research grants from various bodies—such as the National Institute of Health in America or the Australian Research Council—have become increasingly competitive. The reason, as De Peuter and Conix (2022) argue, is because the number of researchers who submit grant applications has increased but the availability of funds has not been boosted to the same extent.
To decide which applicants they will fund, most of these bodies apply an approach called peer review. Specifically
-
a panel of relevant scientists, called reviewers, evaluate and score these proposals
-
the scores of these reviewers are combined, and the proposals are ranked
-
only the applicants that are ranked the highest receive funding
The majority of scientists generally believe that peer review, despite possible flaws, is the best approach to allocate research funding (Philipps, 2021). Yet, scholars in this field have shown that peer review may culminate in a range of concerns and problems.
To resolve this concerns, since around 2000 (e.g., Boyle, 1998; Brezis, 2007; Greenberg, 1998), scholars have advocated the possibility of random grant allocation, sometimes called lottery procedures, to decide which grant submissions should be funded. For example, Greenberg (1998) recommended that funding bodies should allocate a specific percentage of their funds—perhaps 15 to 20%--to a lottery. If applicants were chosen in these lotteries, they would receive the funding they sought, provided their projects were first deemed as respectable. Random grant allocation is assumed to overcome several limitations of peer review—the approach that funding bodies usually apply to allocate grants—such as the steep costs or bias towards research that is not especially novel. Specifically, random grant allocation is not only efficient but can increase the diversity of funded projects and can override some injustices around funding.
Because of these benefits, several funding bodies, such as the New Zealand Health Research Council, the Swiss National Science Foundation, the Austrian Science Fund, and the German Volkswagen Foundation have applied this approach. Other funding bodies, including the Council of Canadian Academies and Royal Danish Academy of Science and Letters, have piloted or plan to pilot this approach. Nevertheless, despite some enthusiasm towards random grant allocation, many scholars criticize this approach (Bedessem, 2020; Reinhart & Schendzielorz, 2020)
Limitations of the peer review approach: Expenses
Compared to other approaches, such as random allocation, the peer review approach is expensive to applicants, to reviewers, and to funding bodies. To illustrate the expense to applicants, Herbert and colleagues (2013) estimated the time that researchers had dedicated to one funding round—the Australian National Health and Medical Research Council in 2012. They estimated the 3727 research proposals would have consumed 550 hours of work, equivalent to AU$66 million in salaries. This value represents 14% of the total fund. Only 21% of the projects received funding.
Admittedly, when researchers construct research proposals, they may receive some benefit, even if these projects are not funded. For example, they need to consider their past research and future aspirations more diligently, sometimes clarifying their ideas, goals, and priorities. Nevertheless, as Gross and Bergstrom (2019) revealed, after estimating the return on these grants, scientists would tend to be significantly more productive to society if they could dedicate more time to productive research rather than to grant applications.
Besides the applicants, the reviewers also dedicate significant time to this approach, also detracting time from more productive activities. For example, as Graves et al. (2011) estimated, about 9% of the costs or expenses of one grant scheme or round of the Australian National Health and Medical Research Council can be ascribed to peer review.
Peer review does not only incur these costs but also increases waste. To illustrate, most funding bodies will distribute most the funds to a limited subset of renowned authors. The researchers that receive the funding, therefore, often earn more research income than needed. Hence, these researchers do not tend to use these funds as effectively (Mongeon et al. 2016). Similarly, according to Wahls (2018), research projects in health that receive about $400k annually from the National Institute of Health are the most efficient. Yet, a few projects receive significantly more, and many projects receive significantly less, than $400k annually.
Limitations of the peer review approach: Limited precision of peer evaluations
Peer reviewers will tend to assign each application or proposal a score. Yet, because peer reviewers depend on intuition and judgment, these scores are not especially precise. If the reviewers evaluated the same applications twice, the scores would inevitably be different.
The problem, however, is that a difference in decimals, such as 3.09 out of 5 and 3.08 out of 5, is often enough to differentiate successful grant applications from unsuccessful grant applications. Indeed, as Kaplan et al (2008) estimated, if the NHS wanted to limit this random error to .01 on a 5-point scale, over 38 000 reviewers would need to be engaged in evaluate the applications. If funding bodies merely wanted to limit this random error to .1 on a 5-point scale, 384 reviewers would still need to be engaged.
Limitations of the peer review approach: Relevance of ratings
To evaluate grant applications, funders usually receive explicit instructions on how to evaluate the various criteria, such as quality, impact, and track record. But whether the scores they assign to each application predict return on investment is contentious. That is, because of several reasons, the criteria do not necessarily culminate in scores that reflect possible quality or return on investment. To illustrate
-
experienced reviewers often deviate from these instructions, because they overestimate their knowledge of peer reviews (Sattler et al. 2015)
-
reviewers cannot predict which research projects are likely to be successful—but instead may derive these predictions from similar projects in the past; hence, biases in the past that favor specific projects will magnify over time (Mallapaty, 2018)
-
typically, the reviewers of grant applications, unlike the reviewers of publications, are seldom leading specialists in the field
Because of these concerns, the scores tend to vary considerably across reviewers. As Gallo et al. (2016) estimated, over 75% of the variance in these scores can be attributed to either random error, variations across reviewers, or the interaction between variation across reviewers and the specific project. Nevertheless, reviewers often concur on which of the projects outstanding (Li & Agha 2015) but not as likely to concur on the quality of other projects
Limitations of the peer review approach: Bias in ratings
Not only are peer evaluations often inaccurate rather than precise and meaningful, the scores are sometimes biased towards particular demographics, even if these biases are unconscious and inadvertent. To illustrate
-
males often receive higher scores, even when gender is concealed, because they tend to use more abstract language (Else 2019; for a nuanced discussion, see Sato et al 2020)
-
reviewers tend to evaluate the same projects more favorably if the track record of applicants is extensive (Guthrie et al. 2019)
-
research that is especially innovative tends to be rated unfavorably partly because the results of innovative studies is harder to predict and partly because innovative projects may not confirm to the conventional standards that reviewers espouse (Brezis 2007; Gillies 2014; Li & Agha 2015)
-
research that integrates multiple disciplines or FOR codes is more likely to be rated unfavorably, because the projects may not conform to the standards the reviewers have adopted (e.g., Bromham et al., 2016).
Limitations of the peer review approach: A potential source of research misconduct
Because peer review is so competitive, this approach might discourage research integrity. To illustrate
-
because reviewers are not compensated but often need to evaluate many applications, they often skim application and thus inflate their confidence in their scores (e.g., Herbert et al 2013)
-
to be competitive, applicants may feel obliged to exaggerate the milestones they can deliver within a specific timeframe (De Peuter & Conix, 2022) or the implications of their research (Serrano Velarde, 2018)
Possible improvements to the peer review approach: Measures to diminish the impact of inconsistent scores
Because of the problems and concerns about the peer review approach, many scholars have recommended entirely novel alternatives—most commonly random grant allocation. However, even smaller changes to the peer review approach could offset or diminish some of these problems.
First, many of the criticisms that are levelled at the peer review approach revolve around the inaccuracy or bias of scores—inaccuracies or biases that often manifest as inconsistencies across reviewers. Therefore, measures that diminish the impact of these inconsistencies might significantly improve the integrity and utility of the peer review approach. To diminish these inconsistencies, several measures have been recommended:
-
reviewers could receive more training (Sattler et al. 2015), although past research implies this approach may not be effective (Pier et al. 2018)
-
the lowest score that is assigned to each applicant should be deleted, to diminish the established impact of low outliers (Daniels, 2015)
-
perhaps variability in reviewer scores could be deemed as an indication the project could be innovative and the returns, as well as risks, could be elevated (Kaplan et al., 2008)
-
the panel of reviewers could also include other relevant stakeholders, such as farmers (Bedessem, 2020)
Possible improvements to the peer review approach: Measures to diminish costs
Scholars have also proposed several measures to diminish the costs and expenses of peer review. For example
-
whether shorter applications save time would need to be investigated empirically
-
an approach that comprises two phases—in which researchers submit a full application only after initial expressions of interest are accepted—has been shown to reduce expense by 30% in particular circumstances (Morgan et al. 2020)
Random grant allocation strategies: An example
To override the problems and limitations of peer evaluations, many scholars have advocated random allocation, at least in specific circumstances. But few funding agencies have implemented this approach.
One of the first exceptions was the Health Research Council of New Zealand (for a discussion, see Liu et al., 2020). Since 2013, random allocation has been applied to one scheme, called the Explorer Grant—a scheme that revolves around transformative research in health. To be eligible, the proposed ideas, methodologies, or technologies must be deemed as transformative, innovative, or unconventional. These applications would not be eligible to receive other Health Research Council funding. Successful applications receive NZD $150,000.
To apply, researchers submit brief applications, comprising six pages or fewer, and need to enter limited administrative information. A panel of three members, selected because of their capacity to think innovatively and their expertise in either biomedical, clinical, or public health, assess each anonymous application on two criteria only:
-
whether the research is potentially transformative rather than merely an incremental refinement to past research
-
whether the research is viable—such as whether the research could be completed
If two or more of the panel members perceive the research as both potentially transformative and viable, the application is included in the lottery. Then, to randomly allocate the applications, the funding agency deploy Microsoft Excel to randomly assign a number to each proposal. The projects that are assigned smaller numbers receive funding, until the funding pool is exhausted. All applicants are informed about this procedure.
Random grant allocation strategies: The perspective of researchers
Presumably, random grant allocation may be applicable in some circumstances but not in other circumstances. Barlösius and Philipps (2022) thus explored the circumstances in which researchers felt that random grant allocation may be useful. In particular, this study examined which the perspective of researchers on which problems this random grant allocation could address. To address this question, Barlösius and Philipps interviewed 32 researchers, ranging from doctoral candidates to professors, specializing in biology or physics.
In general, the researchers felt that random allocation may address a range of problems with peer review. Specifically, random allocation might diminish a range of problems. For example, when funding bodies depend on peer review
-
reviewers often reject the applications of gifted researchers who are not yet renowned
-
reviewers sometimes reject the applications that might challenge their own research or favor researchers in their network
Random allocation can overcome these problems, because the biases of reviewers do not affect the allocation of funding. However, according to the participants, random allocation cannot address some of the other limitations of peer review, such as the problem that
-
members of funding bodies sometimes use the ideas of submitted research proposals
-
the feedback that applicants receive is often inadequate
-
researchers do not know which topics will be prioritized and thus cannot readily choose the issues to explore
More interestingly, according to participants, some of the matters that random allocation may address may not be problems at all but are legitimate features of science. To illustrate, according to participants
-
bold and unconventional research tends to be rejected, diminishing the tendency of researcher to propose daring research projects.
-
reviewers tend to apply their intuition and judgment, rather than a methodical approach, underpinned by science, to rank applicants
Although random allocation may address these matters, the rejection of daring projects and the reliance on reviewer judgment are essential features of scientific progress. For example, this rejection of daring projects, although potentially stifling progress, also confines funding to projects that are more likely to be effective. Likewise, the reliance on judgment and intuition enables reviewers to consider priorities that are hard to characterize in algorithms or formulas.
Because of these considerations, the participants felt the random allocation of grants may be applicable, but only in specific circumstances and if preceded by attempts to confirm the scientific quality of applications. According to participants, random allocation may be suitable when
-
funding bodies want to confine some of their funding to support daring, innovative projects and researchers
-
multiple applications are deemed as equally worthy but only one application can be funded
-
funding bodies want to override the inherent bias towards more established researchers
-
funding bodies need to reach decisions more efficiently.
Random grant allocation strategies: The perspective of applicants
In addition to the attitudes of researchers in general, some research has explored the attitudes of applicants to specific instances of random allocation. To illustrate, the Explorer Grants in New Zealand are allocated randomly, provided the research is deemed to be transformative and viable. Liu et al. (2020) conducted a survey to evaluate the attitudes of applicants towards this scheme. Interestingly, 78% of the applicants who received funding deemed this random allocation is acceptable, whereas only 44% of the applicants who did receive funding deemed this random allocation is acceptable. However, in general, these individuals felt this random allocation is suitable only to grant schemes that revolve around transformational research.
Features of randomized grant allocation: A triage phase
One problem with randomized grant allocation is that research proposals that are patently inadequate might be funded. Conversely, research proposals that are patently outstanding might be rejected. To address this problem, many if not most proponents of randomized grant allocation recommend a preliminary review before the random allocation. This preliminary review might comprise several phases.
First, an exclusive team of reviewers might evaluate the grant applications, but perhaps more expeditiously than typical peer reviews. They might, for example, evaluate only a brief summary (e.g., Gross & Bergstrom, 2019). Second, grant applications that are obviously unsuitable—perhaps because they do not fulfill several minimum criteria or because all reviewers criticized this proposal—would be rejected before the lottery (e.g., Gillies, 2014; Liu et al. 2020). Third, grant applications that are conspicuously outstanding may be funded and bypass the lottery. Finally, all the other grant applications—applications that are not obviously unsuitable or outstanding (Graves et al., 2011) or applications that did not reach consensus (Brezis 2007)—would be submitted to the lottery.
Features of randomized grant allocation: Minimal criteria
Most scholars who advocate random allocation concede that some preliminary activity, such as triage phase, must precede the lottery. Otherwise, anyone could submit an application and receive a grant. The question, then, becomes how should funding agencies decide which applications to withhold from the lottery.
According to Avin (2018), funding agencies should stipulate the minimum criteria that an application must fulfill. To illustrate, funding agencies might stipulate that researchers cannot submit an application unless
-
they have attained a doctoral qualification in institutions that are ranked above a particular threshold
-
they have published at least one paper in one or several relevant journals, and so forth
To develop these minimum criteria, Avin (2018) recommends that
-
researchers should be able to pursue multiple pathways to fulfill each criterion; otherwise, particular segments of the population might be unfairly disadvantaged
-
these criteria should be discussed and refined regularly and collaboratively with all relevant stakeholders
Features of randomized grant allocation: Short proposals
If proposals merely need to fulfill minimum criteria to be included in a lottery, the proposals do not need to be lengthy. According to Avin (2018), proposals should be detailed enough only to fulfil four purposes:
-
The proposal should clarify the topic, to enable the funding body to distribute this submission to the right panel
-
The authors should demonstrate knowledge of some relevant literature; even radical proposals should be informed by some past knowledge
-
The proposal should include enough detail to enable reviewers to ascertain promptly whether the merit of this research is broadly likely to be high, moderate, low, or uncertain; this information could be used to identify applications that should circumvent the lottery or be rejected outright.
Features of randomized grant allocation: Graduated lottery
Most random allocation schemes assume that applications are either included or excluded from the lottery. However, funding bodies could use a graduated lottery, foreshadowed by Boyle (1998) and suggested by De Peuter and Conix (2022). To illustrate, according to this scheme
-
the grant applications would be ranked, at least cursorily
-
half the applications in the lowest quartile would be randomly selected
-
the selected applications would be combined with the next lowest quartile—and half this set of applications would be randomly selected
-
this procedure would be extended to all four quartiles
The benefit of this scheme is that applicants, initially evaluated favorably, would be more likely funded. However, this scheme does not depend excessively on the evaluations of reviewers
Limitations of random allocation: Circumstances in which randomized allocation may not be appropriate
Most scholars recognize that randomized allocation of research grants may be more applicable in some circumstances than other circumstances. Avin (2018) discussed some of the circumstances in which randomized allocation may be unsuitable:
-
Randomized allocation should not be applied to fund massive projects—like the Human Genome Project—that need to receive millions of dollars over decades. No project that is only randomly selected should receive decades of funding
-
Randomized allocation is not as likely to be effective when a funding agency wants to encourage research that solves a specific problem, such as an epidemic
References
-
Avin, S. (2018). Policy considerations for random allocation of research funds. RT. A Journal on Research Policy and Evaluation, 6(1).
-
Barlösius, E., & Philipps, A. (2022). Random grant allocation from the researchers’ perspective: Introducing the distinction into legitimate and illegitimate problems in Bourdieu’s field theory. Social Science Information, 61(1), 154-178.
-
Bedessem, B. (2020). Should we fund research randomly? An epistemological criticism of the lottery model as an alternative to peer review for the funding of science. Research Evaluation, 29(2), 150-157.
-
Bornmann, L., & Daniel, H. D. (2009). The luck of the referee draw: The effect of exchanging reviews. Learned Publishing, 22(2), 117-125.
-
Boyle, C. (1998). Organizations selecting people: how the process could be made fairer by the appropriate use of lotteries. Journal of the Royal Statistical Society: Series D (The Statistician), 47(2), 291-321.
-
Brezis, E. S. (2007). Focal randomisation: An optimal mechanism for the evaluation of R&D projects. Science and Public Policy, 34(10), 691-698.
-
Bromham, L., Dinnage, R., & Hua, X. (2016). Interdisciplinary research has consistently lower funding success. Nature, 534(7609), 684-687.
-
Chawla, D. S. (2021). Swiss funder draws lots to make grant decisions. Nature.
-
Cole, S., Cole, J. R., & Simon, G. A. (1981). Chance and consensus in peer review. Science, 214(4523), 881-886.
-
Daniels, R. J. (2015). A generation at risk: young investigators and the future of the biomedical workforce. Proceedings of the National Academy of Sciences, 112(2), 313-318.
-
De Peuter, S., & Conix, S. (2022). The modified lottery: Formalizing the intrinsic randomness of research funding. Accountability in Research, 29(5), 324-345.
-
Else, H. (2019). Male researchers''vague'language more likely to win grants. Nature.
-
Fang, F. C., & Casadevall, A. (2016). Research funding: The case for a modified lottery. MBio, 7(2), e00422-16.
-
Gallo, S. A., Sullivan, J. H., & Glisson, S. R. (2016). The influence of peer reviewer expertise on the evaluation of research funding applications. PloS one, 11(10).
-
Gildenhuys, P. (2020). Lotteries make science fairer. Journal of Responsible Innovation, 7(sup2), S30-S43.
-
Gillies, D. (2014). Selecting applications for funding: Why random choice is better than peer review. RT. A Journal on Research Policy and Evaluation, 2(1).
-
Graves, N., Barnett, A. G., & Clarke, P. (2011). Funding grant proposals for scientific research: retrospective analysis of scores by members of grant review panel. BMJ, 343.
-
Greenberg, D. S. (1998). Chance and grants. The Lancet, 351(9103), 686.
-
Gross, K., & Bergstrom, C. T. (2019). Contest models highlight inherent inefficiencies of scientific funding competitions. PLoS Biology, 17(1)
-
Guthrie, S., Rincon, D. R., McInroy, G., Ioppolo, B., & Gunashekar, S. (2019). Measuring bias, burden and conservatism in research funding processes. F1000Research, 8(851).
-
Herbert, D. L., Barnett, A. G., Clarke, P., & Graves, N. (2013). On the time spent preparing grant proposals: an observational study of Australian researchers. BMJ open, 3(5), e002800.
-
Höylä, T., Bartneck, C., & Tiihonen, T. (2016). The consequences of competition: Simulating the effects of research grant allocation strategies. Scientometrics, 108(1), 263-288.
-
Kaplan, D., Lacetera, N., & Kaplan, C. (2008). Sample size and precision in NIH peer review. PLoS One, 3(7), e2761.
-
Langfeldt, L. (2001). The decision-making constraints and processes of grant peer review, and their effects on the review outcome. Social Studies of Science, 31(6), 820-841.
-
Lee, C. J., Grant, S., & Erosheva, E. A. (2020). Alternative grant models might perpetuate Black–White funding gaps. The Lancet, 396(10256), 955-956.
-
Li, D., & Agha, L. (2015). Big names or big ideas: Do peer-review panels select the best science proposals? Science, 348(6233), 434-438.
-
Liu, M., Choy, V., Clarke, P., Barnett, A., Blakely, T., & Pomeroy, L. (2020). The acceptability of using a lottery to allocate research funding: a survey of applicants. Research Integrity and Peer Review, 5(1), 1-7.
-
Luukkonen, T. (2012). Conservatism and risk-taking in peer review: Emerging ERC practices. Research Evaluation, 21(1), 48-60.
-
Magua, W., Zhu, X., Bhattacharya, A., Filut, A., Potvien, A., Leatherberry, R., ... & Kaatz, A. (2017). Are female applicants disadvantaged in National Institutes of Health peer review? Combining algorithmic text mining and qualitative methods to detect evaluative differences in R01 reviewers' critiques. Journal of women's health, 26(5), 560-570.
-
Mongeon, P., Brodeur, C., Beaudry, C., & Larivière, V. (2016). Concentration of research funding leads to decreasing marginal returns. Research Evaluation, 25(4), 396-404.
-
Morgan, B., Yu, L. M., Solomon, T., & Ziebland, S. (2020). Assessing health research grant applications: A retrospective comparative review of a one-stage versus a two-stage application assessment process. Plos one, 15(3), e0230118.
-
Pier, E. L., Brauer, M., Filut, A., Kaatz, A., Raclaw, J., Nathan, M. J., ... & Carnes, M. (2018). Low agreement among reviewers evaluating the same NIH grant applications. Proceedings of the National Academy of Sciences, 115(12), 2952-2957.
-
Philipps, A. (2021). Science rules! A qualitative study of scientists’ approaches to grant lottery. Research Evaluation, 30(1), 102-111.
-
Reinhart, M., & Schendzielorz, C. (2020). The lottery in Babylon—On the role of chance in scientific success. Journal of Responsible Innovation, 7, S25-S29.
-
Roumbanis, L. (2019). Peer review or lottery? A critical analysis of two different forms of decision-making mechanisms for allocation of research grants. Science, Technology, & Human Values, 44(6), 994-1019.
-
Roumbanis, L. (2020). Two dogmas of peer-reviewism. Journal of Responsible Innovation, 7(sup2), S129-S133.
-
Roumbanis, L. (2021). Disagreement and agonistic chance in peer review. Science, Technology, & Human Values.
-
Sato, S., Gygax, P. M., Randall, J., & Schmid Mast, M. (2021). The leaky pipeline in research grant peer review and funding decisions: challenges and future directions. Higher Education, 82(1), 145-162.
-
Sattler, D. N., McKnight, P. E., Naney, L., & Mathis, R. (2015). Grant peer review: improving inter-rater reliability with training. PloS one, 10(6), e0130450.
-
Serrano Velarde, K. (2018). The way we ask for money… The emergence and institutionalization of grant writing practices in academia. Minerva, 56(1), 85-107.
-
Wahls, W. P. (2018). Point of view: The NIH must reduce disparities in funding to maximize its return on investments from taxpayers. Elife, 7, e34965.